Model Context Protocol(MCP) 是一个专为大型语言模型(LLM)设计的协议,旨在通过标准化接口为 LLM 提供动态上下文、工具和提示,从而增强 AI 应用的灵活性和实用性。本文将深入探讨 MCP 服务开发的细节,包括核心组件、开发流程、代码示例、实际用例以及注意事项,帮助开发者快速上手并构建强大的 MCP 服务。
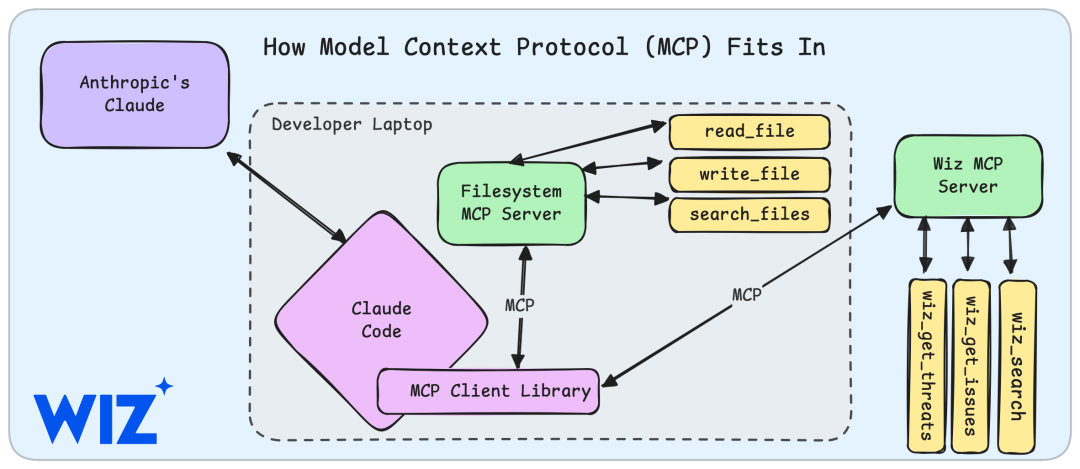
什么是 MCP?
MCP(Model Context Protocol)是一个由服务器端实现的协议,允许客户端(通常是 LLM 或支持 LLM 的应用)通过标准化的 URI 访问 Resources(资源)、调用 Tools(工具) 和使用 Prompts(提示)。其目标是为 LLM 提供外部上下文和功能,弥补模型在实时数据、特定领域知识或复杂操作上的局限。
可以参考之前的文章:
探索模型上下文协议(MCP):大型语言模型与外部世界的桥梁
一文精讲 – MCP与LLM函数调用的区别
MCP 的三大核心组件
1.Resources(资源):•只读数据,如文件内容、API 响应或数据库记录。•通过 URI(如 file:///path/to/file
或 weather://{city}
)访问。•用途:为 LLM 提供上下文,如合同文件、实时天气数据。2.Tools(工具):•可执行的操作或函数,如发送请求、执行计算。•需要用户或 LLM 批准调用。•用途:实现复杂功能,如调用外部 API 或修改数据。3.Prompts(提示):•预定义的模板,指导 LLM 完成特定任务,如翻译、总结。•通过 URI(如 prompt://summarize
)访问。•用途:标准化 LLM 的输出格式或行为。
MCP 的工作机制
•服务器端:使用 MCP SDK(如 Python 的 FastMCP
)定义 Resources、Tools 和 Prompts,并通过 HTTP 服务器暴露。•客户端:LLM 或支持 MCP 的应用(如 Claude Desktop、Cursor)通过 HTTP 请求(GET/POST)访问服务器提供的 URI。•交互:客户端将 Resource 数据或 Tool 结果注入 LLM 上下文,Prompt 指导 LLM 生成最终输出。
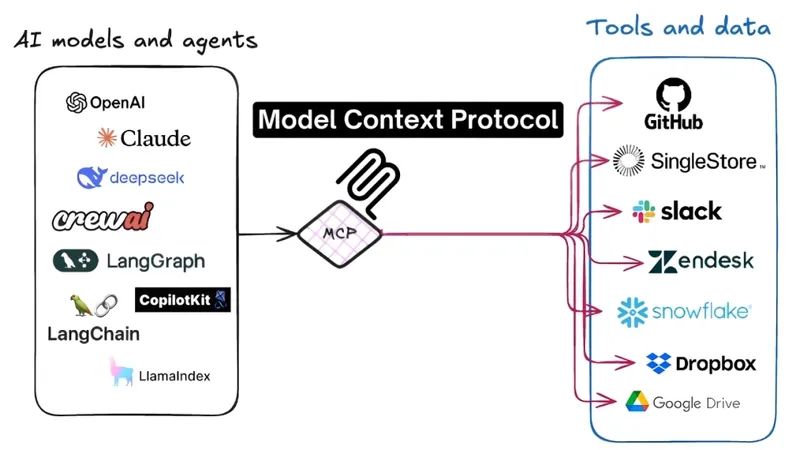
MCP 服务开发流程
开发 MCP 服务主要分为以下步骤:
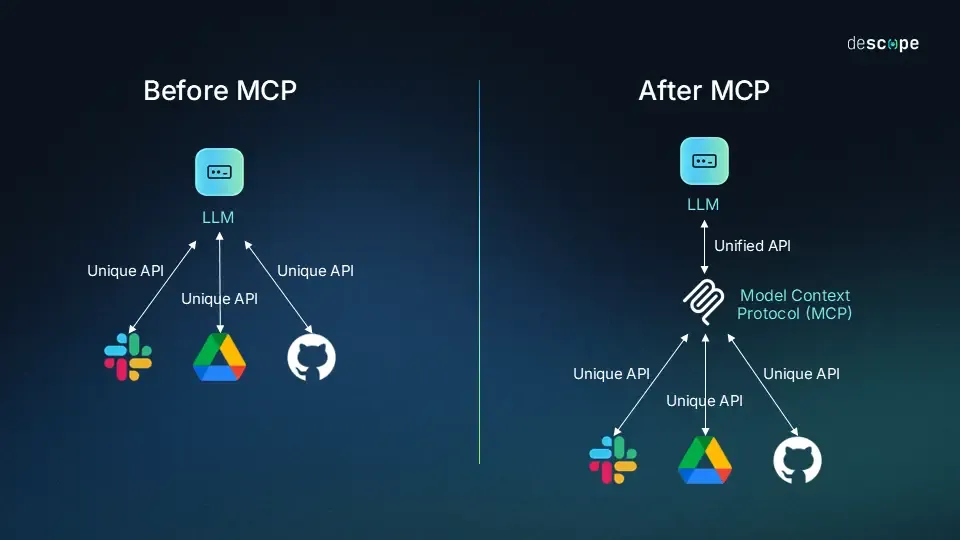
1. 环境搭建
•安装 Python 和 MCP SDK: 推荐使用 Python 3.8+ 和 uv
工具管理虚拟环境:
pip install uvuv venv
source .venv/bin/activate
uv pip install mcp[cli]
mcp --version
2. 创建 MCP 服务
使用 Python 的 FastMCP
库快速搭建服务。以下是一个基础示例:
from mcp.server.fastmcp importFastMCP
# 初始化 MCP 实例
mcp =FastMCP("DemoApp")
# 定义一个 Resource
def greet(name: str)-> str:
return f"Hello, {name}!"
# 定义一个 Tool
def calculate_sum(a:int, b:int)->int:
return a + b
# 定义一个 Prompt
def summarize()-> str:
return"Please summarize the following content in 100 words or less: {content}"
# 启动服务
if __name__ =="__main__":
print("Starting MCP server...")
mcp.run(host="0.0.0.0", port=8000)
说明:
•@mcp.resource
定义只读数据,支持动态参数(如 {name}
)。•@mcp.tool
定义可执行函数,参数需明确类型。•@mcp.prompt
定义提示模板,支持占位符。•mcp.run()
启动 HTTP 服务器,默认监听 localhost:8000
。
3. 测试 MCP 服务
使用 MCP CLI 或 curl
测试服务是否正常:
•列出可用 Resources:
curl http://localhost:8000/resources/list
["greeting://{name}"]
•读取 Resource:
curl http://localhost:8000/resources/read?uri=greeting://Alice
输出:{"content": "Hello, Alice!", "mime_type": "text/plain"}
curl -X POST http://localhost:8000/tools/call \-H "Content-Type: application/json" \-d '{"tool": "calculate_sum", "args": {"a": 5, "b": 3}}'
输出:{"result": 8}
curl http://localhost:8000/prompts/read?uri=summarize
输出:{"content": "Please summarize the following content in 100 words or less: {content}", "mime_type": "text/plain"}
4. 客户端集成
客户端(如 Claude Desktop、Cursor 或自定义应用)通过 HTTP 请求或 MCP SDK 访问服务:
•Python 客户端示例:
from mcp.client importMCPClientclient =MCPClient(host="localhost", port=8000)# 读取 Resourceresource = client.read_resource("greeting://Alice")print(resource.content)# 输出: Hello, Alice!# 调用 Toolresult = client.call_tool("calculate_sum",{"a":5,"b":3})print(result)# 输出: 8# 获取 Promptprompt = client.read_prompt("summarize")print(prompt.content)# 输出: Please summarize...
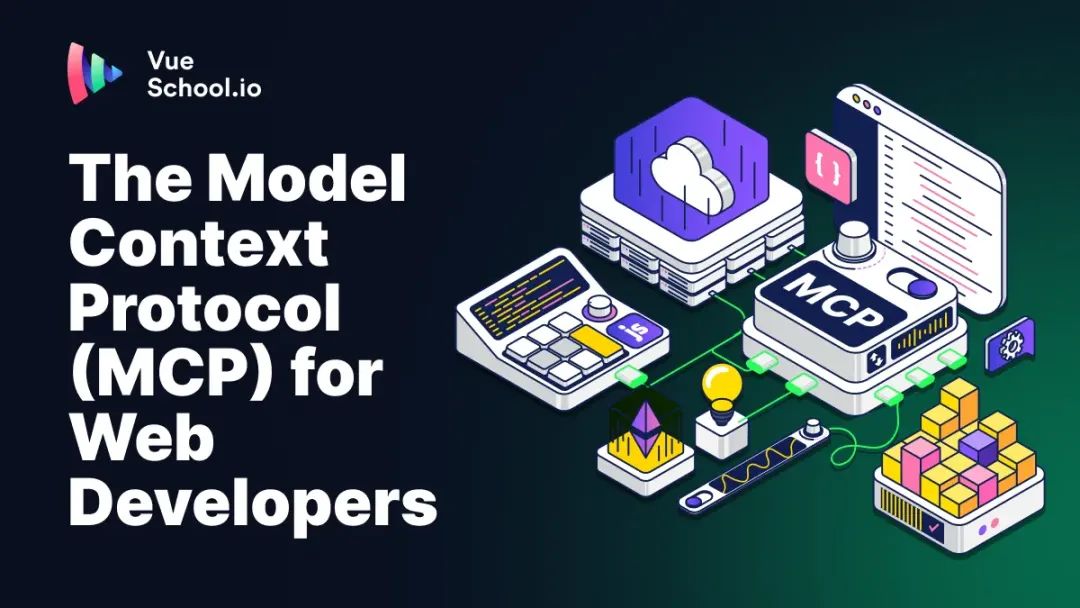
5. 部署与优化
•部署:使用 Docker 或云服务(如 AWS、GCP)部署 MCP 服务器,确保高可用性。•优化:•缓存:对频繁访问的 Resource 使用缓存(如 Redis)。•分块传输:大文件 Resource 支持 range
参数。•安全:通过 OAuth 或 API Token 保护敏感 Resource 和 Tool。
深入剖析核心组件开发
Resources 开发
Resources 是 MCP 的核心,用于为 LLM 提供上下文数据。以下是一个复杂 Resource 示例,展示如何集成外部 API:
import requests
from mcp.server.fastmcp importFastMCP
mcp =FastMCP("WeatherApp")
def get_weather(city: str)-> str:
api_key ="your_api_key"
url = f"https://api.openweathermap.org/data/2.5/weather?q={city}&appid={api_key}"
response = requests.get(url)
data = response.json()
return f"Weather in {city}: {data['weather'][0]['description']}, {data['main']['temp']}K"
if __name__ =="__main__":
mcp.run()
用途:客户端触发 weather://Shanghai
,获取实时天气数据,LLM 基于此生成天气预报。
注意:
•使用 MIME 类型(如 application/json
)明确数据格式。•支持动态 URI 参数(如 {city}
)。•处理错误(如 API 失败)并返回用户友好的消息。
Tools 开发
Tools 提供可执行功能,常用于复杂操作。以下是一个 Tool 示例,结合 Resource:
from mcp.server.fastmcp importFastMCP
mcp =FastMCP("DemoApp")
def get_data(id: str)-> str:
return f"Data for ID {id}: Example content"
def process_data(id: str)-> str:
resource = mcp.read_resource(f"data://{id}")
return f"Processed: {resource.content.upper()}"
if __name__ =="__main__":
mcp.run()
用途:LLM 调用 process_data
Tool,间接触发 data://{id}
Resource,生成处理后的结果。
Prompts 开发
Prompts 标准化 LLM 的行为。以下是一个动态 Prompt 示例:
from mcp.server.fastmcp importFastMCP
mcp =FastMCP("DemoApp")
def translate(lang: str)-> str:
return f"Translate the following text to {lang}: {{content}}"
if __name__ =="__main__":
mcp.run()
用途:客户端触发 translate://French
,LLM 使用该 Prompt 将文本翻译成法语。
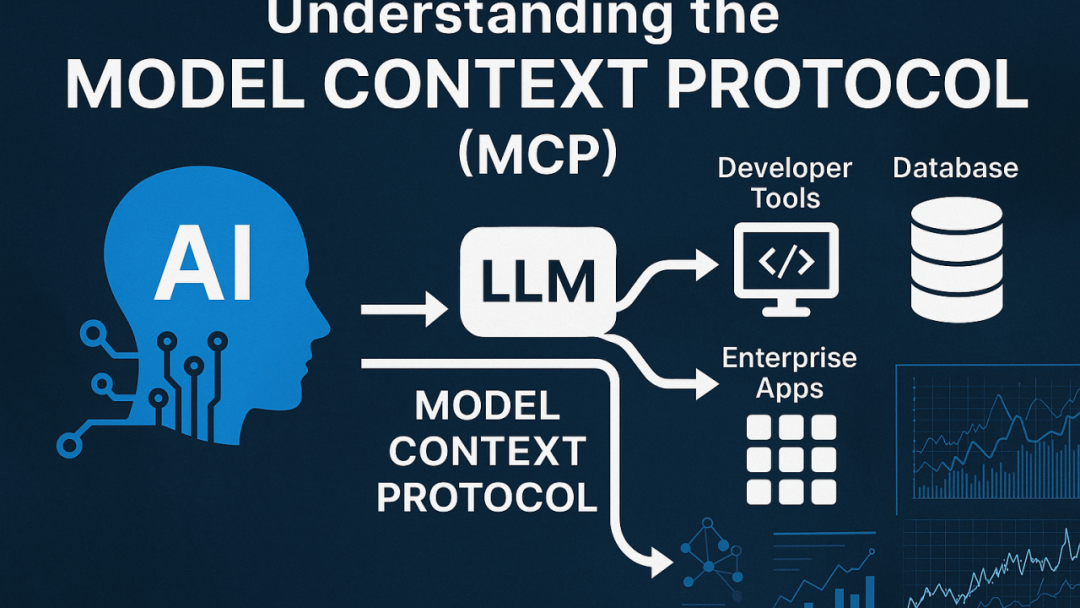
实际用例
1.企业知识库:•场景:公司内部 AI 使用 Resource(如 kb://{article_id}
)访问 Wiki 文档。•实现:定义 Resource 返回 Markdown 内容,LLM 基于此回答员工问题。•代码:
weather://{city}
)获取实时数据。•实现:客户端自动触发 Resource,LLM 生成天气预报。3.文档分析:•场景:法律助手通过 Resource(如 file:///contract.pdf
)读取合同。•实现:客户端将 PDF 内容注入 LLM,生成条款总结。开发注意事项
1.URI 设计:•确保 URI 语义化且唯一(如 file:///path
、weather://{city}
)。•避免冲突,使用命名空间(如 app_name://resource
)。2.性能优化:•大文件 Resource 使用分块传输。•频繁访问的 Resource 使用缓存。3.安全性:•敏感 Resource 和 Tool 需要认证(如 Bearer Token)。•限制客户端访问范围。4.调试:•使用 mcp resources read --uri <uri>
测试 Resource。•启用日志(mcp.run(debug=True)
)排查问题。5.兼容性:•确保 MIME 类型与客户端兼容。•测试不同客户端(如 Claude Desktop、Cursor)。
总结
MCP 服务开发通过 Resources、Tools 和 Prompts 三大组件,为 LLM 提供动态上下文和功能,广泛应用于企业知识库、实时数据查询、文档分析等场景。开发者可以使用 Python 的 FastMCP
快速搭建服务,通过标准化 URI 和 HTTP 接口实现客户端集成。关键在于设计清晰的 URI、优化性能和确保安全性。
希望本文能帮助你深入理解 MCP 服务开发的细节!如果想进一步探讨特定用例或需要更多代码示例,请随时留言。
(文:PyTorch研习社)