
在当今快速发展的 AI 领域,本地运行大型语言模型(LLM)的需求日益增长。llama.cpp 是一个高性能的 C/C++ 库,专门用于运行 LLM,支持多种硬件加速选项。本文将详细介绍如何使用 llama.cpp 在本地运行 DeepSeek-R1 蒸馏模型(1.5B-GGUF),帮助您在消费级硬件上实现高效推理。
一、环境准备
在开始之前,需要确保开发环境已经准备好。这包括安装必要的工具和库,以及配置好适合的硬件环境。
1.下载llama.cpp
首先,需要从 GitHub 克隆 llama.cpp 仓库。llama.cpp 提供了简洁的 API 和高效的内存管理,非常适合在本地运行复杂的模型。
git clone https://github.com/ggerganov/llama.cpp
cd llama.cpp
执行如下:
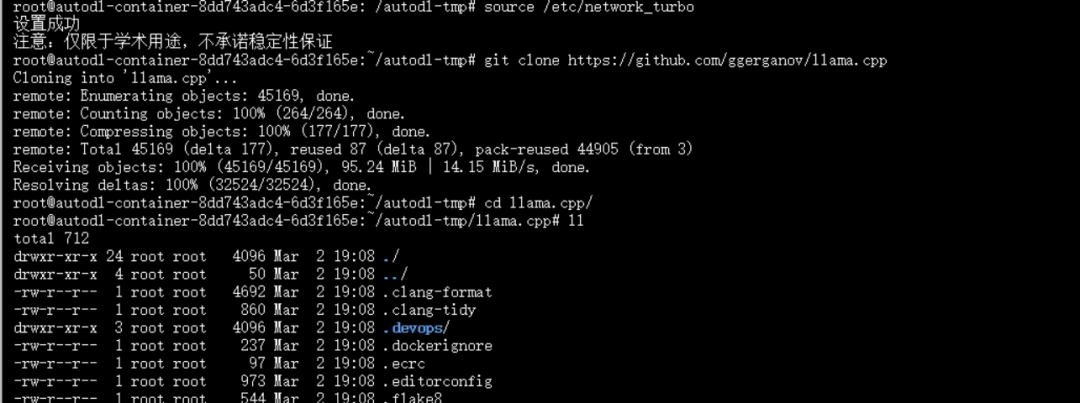
2. 编译llama.cpp
根据您的硬件配置,选择合适的编译命令。`llama.cpp`支持CPU和GPU两种运行模式,您可以根据需求选择。
CPU 构建(适用于无GPU环境):
如果您的设备没有GPU,或者您希望仅使用CPU进行推理,可以使用以下命令进行编译:
cmake -B build
cmake --build build --config Release
这将生成适用于CPU的可执行文件。
GPU 构建(支持CUDA加速):
如果您的设备支持CUDA,并且您希望利用GPU加速模型推理,可以使用以下命令:
cmake -B build -DGGML_CUDA=ON
cmake --build build --config Release
执行如下:
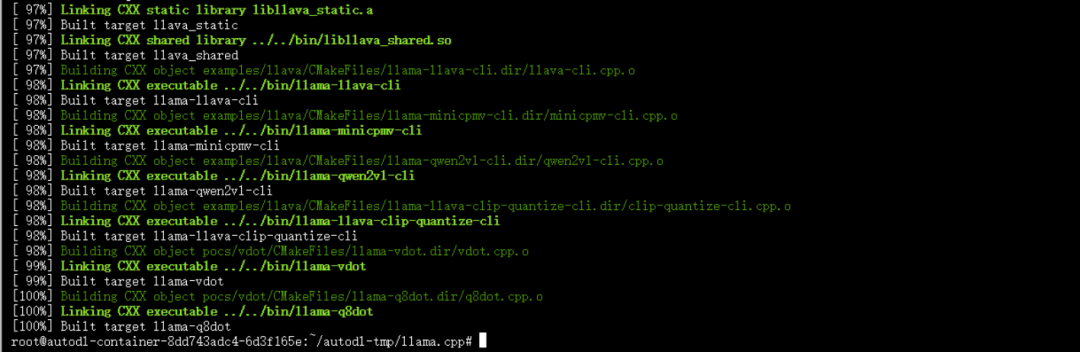
3. 安装依赖
为了运行模型并使用相关的功能,您需要安装一些Python依赖。这些依赖将帮助您下载模型文件,并在Python环境中与模型进行交互。
# 使用`modelscope`库可以方便地下载模型文件,我们需要先安装modelscope:
pip install modelscope
#安装openai,方便后面代码使用openai风格的API接口
pip install openai==0.28
在运行模型之前,需要获取模型文件。`DeepSeek-R1`蒸馏模型是一个经过优化的版本,适合在本地运行,同时保持较高的推理性能。
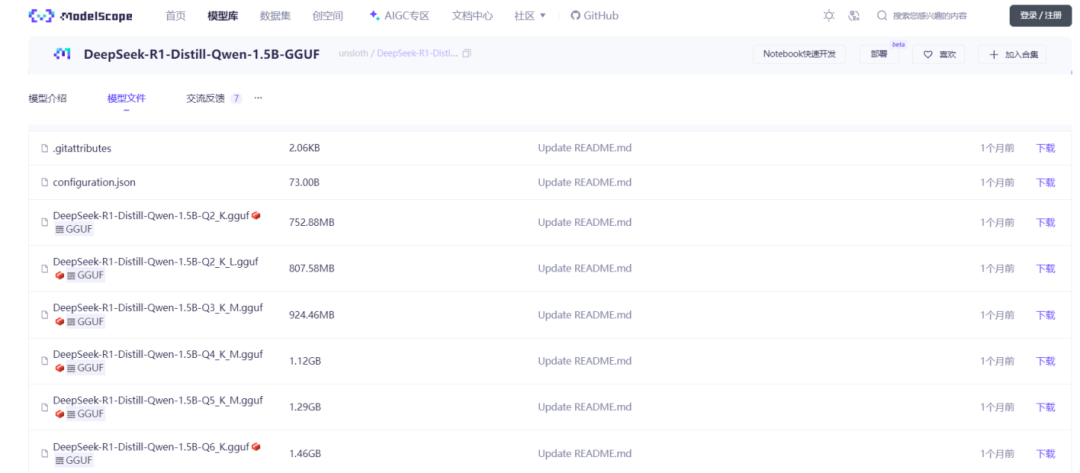
我们从modelscope仓库下载DeepSeek-R1蒸馏版模型。下面我们以Q6_K量化为例,展示如何下载模型文件。
modelscope download --model unsloth/DeepSeek-R1-Distill-Qwen-1.5B-GGUF DeepSeek-R1-Distill-Qwen-1.5B-Q6_K.gguf --local_dir /root/autodl-tmp/models
下载完成后,模型文件将保存到`models`目录。
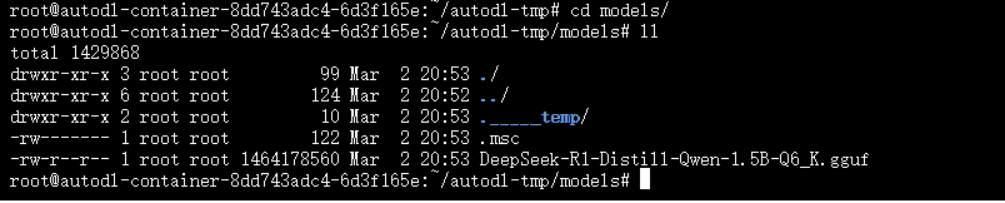
直接下载:如果你喜欢直接下载文件,可以通过以下链接手动下载GGUF文件,并将其保存到`models`目录:
二、启动模型服务(一键启动)
在下载并准备好了模型文件之后,接下来需要启动模型服务。`llama.cpp`提供了一个轻量级的HTTP服务器,可以方便地运行和访问模型。
根据您的操作系统和硬件配置,选择合适的命令启动模型服务:
-
Windows示例(使用预编译二进制):
如果您使用的是Windows系统,并且已经下载了预编译的二进制文件,可以使用以下命令启动服务器:
start cmd.exe /k "llama-server.exe -m models/DeepSeek-R1-Distill-Qwen-1.5B-Q6_K.gguf -c 15000 -ngl 999"
-
Linux/Mac示例(源码编译后):
如果您使用的是Linux或Mac系统,并且已经从源码编译了`llama.cpp`,可以使用以下命令启动服务器:
./build/bin/llama-server -m /root/autodl-tmp/models/DeepSeek-R1-Distill-Qwen-1.5B-Q6_K.gguf --port 8080 -ngl 40
启动完成如下:
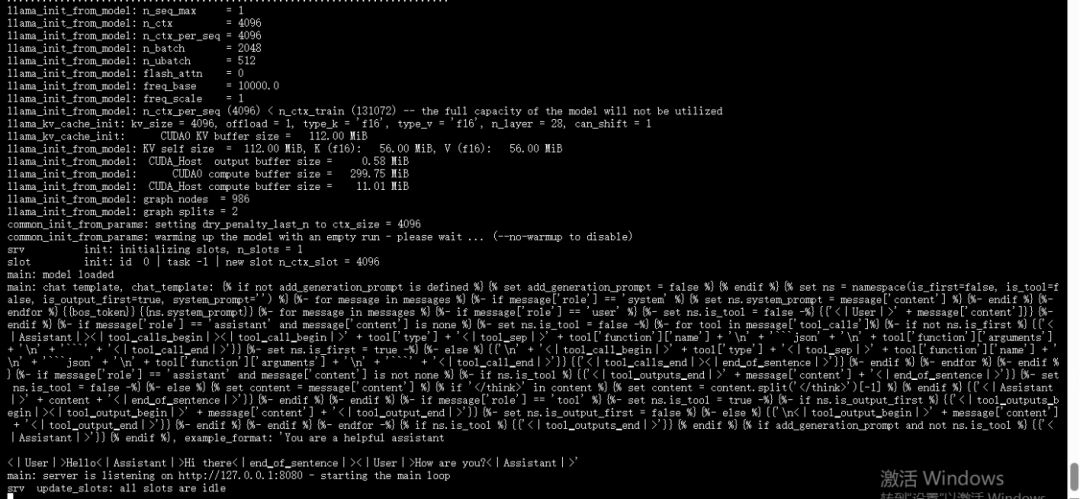
关键参数说明:
-
`-c 15000`:设置上下文长度(根据内存调整)。上下文长度决定了模型在推理时可以处理的最大文本长度。
-
`-ngl 999`:设置GPU层数(设为`0`则纯CPU运行)。如果您希望利用GPU加速,可以根据您的硬件配置调整这个参数。
-
`–host 127.0.0.1`:绑定本地端口。这将使服务器仅在本地运行,确保安全性。
在启动服务后,您可以通过以下方式验证模型是否正常运行
使用验证(一):浏览器界面体验
打开浏览器并访问`http://127.0.0.1:8080`,您应该能够看到一个简单的Web界面,用于测试模型的推理功能。
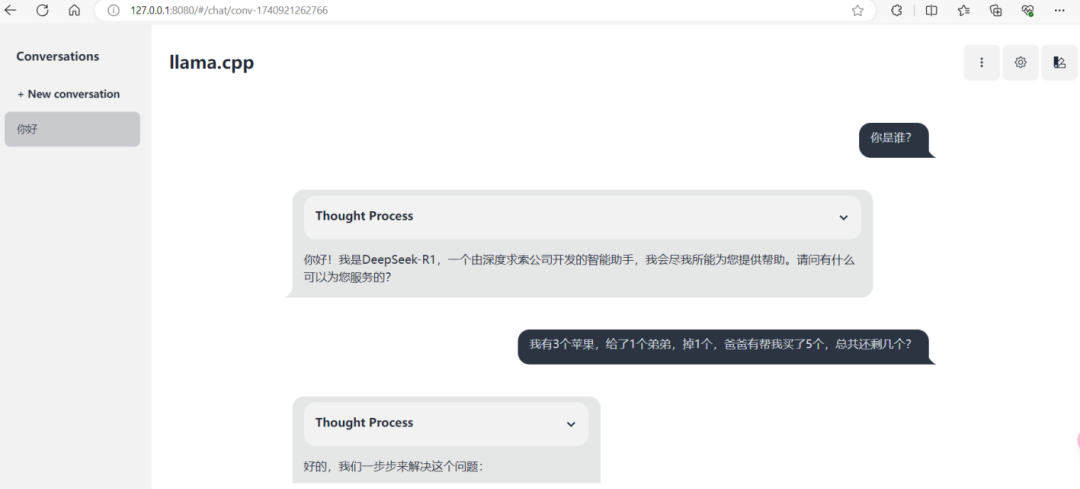
使用验证(二):bash脚本调用API
在命令行窗口,可以使用以下脚本:
curl http://localhost:8080/v1/chat/completions \
-H "Content-Type: application/json" \
-d '{
"model": "DeepSeek-R1-Distill-Qwen-1.5B",
"messages": [{"role": "user", "content": "请介绍一下deepseek"}],
"temperature": 0
}'
模型返回如下:
{"choices":[{"finish_reason":"stop","index":0,"message":{"role":"assistant","content":"<think>\n\n</think>\n\n深度求索人工智能基础技术研究有限公司(简称“深度求索”或“DeepSeek”),成立于2023年,是一家专注于实现AGI的中国公司。"}}],"created":1740921610,"model":"DeepSeek-R1-Distill-Qwen-1.5B","system_fingerprint":"b4798-1782cdfe","object":"chat.completion","usage":{"completion_tokens":42,"prompt_tokens":7,"total_tokens":49},"id":"chatcmpl-cmfV78hIDgvBQkL1kh6LivxpthPOgA3p","timings":{"prompt_n":5,"prompt_ms":24.283,"prompt_per_token_ms":4.8566,"prompt_per_second":205.90536589383518,"predicted_n":42,"predicted_ms":173.381,"predicted_per_token_ms":4.128119047619047,"predicted_per_second":242.24107601178906}}
使用验证(三):Python脚本调用API
在Python环境中使用模型,可以使用以下脚本:
import openai
openai.api_base = "http://127.0.0.1:8080/v1"
openai.api_key = "EMPTY" # Not used
response = openai.Completion.create(
model="local",
prompt="What is 1+1?"
)
print(response.choices[0].text)
这将调用模型并输出推理结果,如下:
*and* 1+1.
Wait, no, that's just one question. Maybe I should try another approach. Wait, perhaps I'm overcomplicating things.
Actually, I need to solve the equation: 1+1=10 in some base. Hmm, okay, so let's think about number bases. In base 10, we know that 1+1=2. But in other bases, the representation changes. So, if I have the equation 1+1=10 in some base, what would that base be?
Wait, so 10 in base b is equal to 1*b + 0 = b in base 10. Similarly, 1+1=2 in base 10. So, in the equation, 1+1=10 in base b translates to 2 = b in base 10. So, solving for b, we get b=2. So, the base is 2. Hmm, that seems straightforward, but maybe I'm missing something.
Alternatively, maybe the equation isn't 1+1=10 in base b, but perhaps 1+1=10 in base 10? No, that doesn't make sense because in base 10, 1+1=2, which isn't 10. So, that can't be. So, I think my initial reasoning is correct.
But just to double-check, let's see: if in base b, 1+1 equals 10, then in base 10, this would mean 1*b + 1 = 1*b + 0? Wait, no, 10 in base b is equal to 1*b + 0. So, 1+1=2, but 10 in base b is equal to b. So, 2 must equal b. Therefore, b is 2.
But, just to make sure I haven't overlooked something, let me think about another approach. In base b, the digits must satisfy 0 ≤ digit < b. So, in the equation 1+1=10, the digits are 1,1, and 0. In base 2, the digits can only be 0 or 1, which is fine because the digits in the equation are 1 and 0. So, base 2 is acceptable.
Another way to think about it: when adding 1 and 1 in base b, if the result is 10, which is equal to b in base 10. So, since 1+1=2 in base 10, that must be equal to b. Therefore, b=2.
Alternatively, maybe we can consider that in base b, the number 10 represents b in base 10. So, 1+1=2, and in base b, 2 must equal 10, meaning that 2= b. Hence, b=2.
So, after thinking through different angles, I'm confident that the base is 2.
**Final Answer**
The base is \boxed{2}.
</think>
To solve the equation \(1 + 1 = 10\) in some base, we need to determine the base \(b\) such that the equation holds true.
1. In any base \(b\), the number \(10\) represents \(b\) in base 10.
2. The equation \(1 + 1 = 10\) translates to \(1 + 1 = b\) in base 10.
3. Simplifying this, we get \(2 = b\).
Thus, the base \(b\) is 2. This is confirmed because in base 2, the number \(10\) represents 2 in base 10, and the addition \(1 + 1\) in base 2 results in 10.
Therefore, the base is \(\boxed{2}\).
三、性能优化与问题排查
在运行模型时,您可能会遇到一些性能问题或错误。以下是一些优化建议和常见问题的解决方案:
1. 硬件适配建议
-
显存不足:如果您遇到显存不足的问题,可以尝试降低`-ngl`值(减少GPU层数),或者使用低量化版本(如`Q4_K_M`)。低量化版本可以在减少内存占用的同时,保持较高的推理性能。
-
内存限制:对于32GB内存的设备,建议运行`Q4`量化版。1.5B模型至少需要8GB内存。如果您的设备内存较小,建议选择更小的模型版本。
2. 常见错误解决
-
Tokenizer错误:如果您遇到Tokenizer相关的错误,请确保`llama.cpp`版本不低于`b4514`。更新到最新版本通常可以解决这类问题。
-
模型加载失败:如果模型加载失败,请检查GGUF文件的完整性,或者尝试重新下载模型文件。确保下载的文件与您选择的模型版本一致。
四、总结
通过`llama.cpp`运行DeepSeek-R1蒸馏版模型,您可以在消费级硬件上体验高性能推理。`llama.cpp`提供了灵活的配置选项,支持多种硬件加速方式,并且易于部署。建议优先使用预编译二进制文件以简化部署流程,并根据硬件配置调整量化参数与GPU层数。如果您需要更高的推理精度,可以尝试运行更大参数的模型;希望本指南能帮助您顺利运行DeepSeek-R1模型,享受高效、灵活的本地推理体验!
(文:小兵的AI视界)